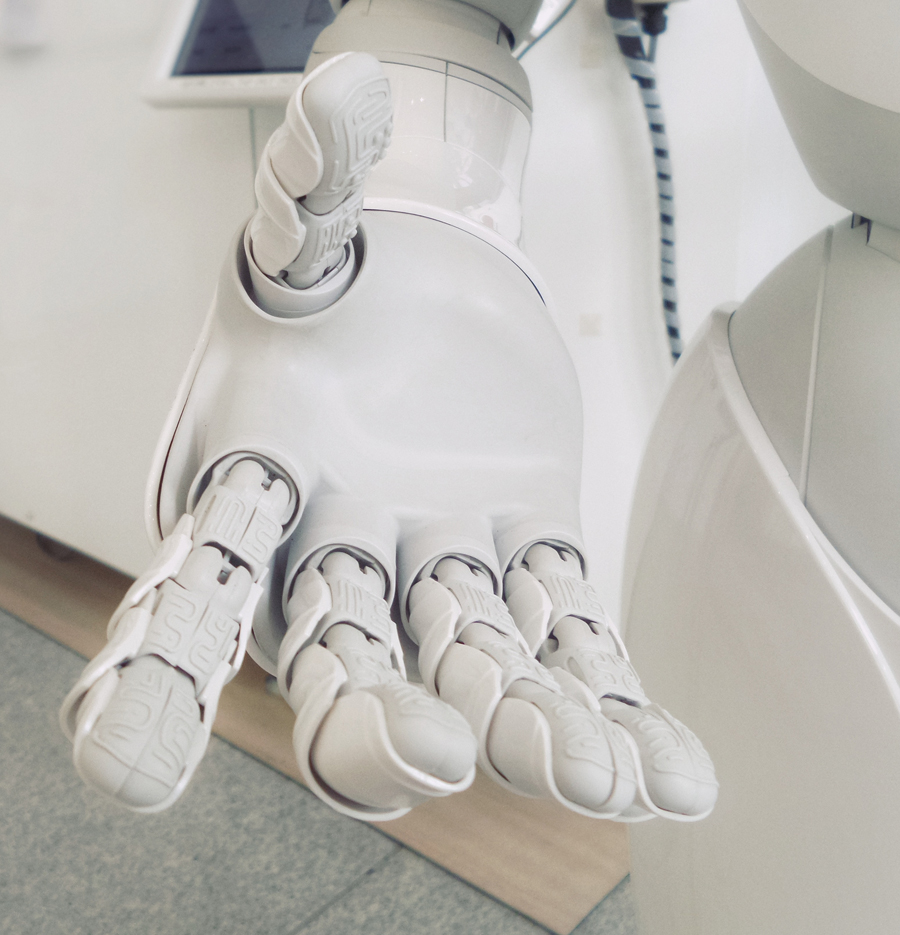
Even very young babies are able to recognise that other people’s hands are similar to their mothers’ and so can be expected to perform the same activities and functions, such as picking them up or feeding them from a bottle.
That’s because humans come to understand, from an early age, the kinematic properties of parts of the body such as hands – their appearance and their ability to move – explains Dr Hyung Jin Chang, an expert in human-centred visual learning in the School of Computer Science's Intelligent Robotics Laboratory. Crucially, youngsters realise hands are hands even if their size or colour are different.
If robots are to become more human-like, they need to be able to do the same thing. For example, programming a robot to cut up a piece of paper with scissors not only requires it to visualise paper and understand cutting techniques, it also needs to recognise that although a small, slim pair of scissors might look different to a large, chunky pair, they have similar properties and therefore fulfil the same function.
Hyung Jin discusses this topic in his paper entitled ‘Highly Articulated Kinematic Structure Estimation Combing Motion and Skeleton Information,’ published in September 2018 in the IEEE Transactions on Pattern Analysis and Machine Intelligence. Co-written with his former colleague at Imperial College London, Professor Yiannis Demiris, it was recently awarded the College’s Paper of the Month accolade.
‘The human is at the centre of my interest in robotics: learning from and about humans are two of the main themes of my work,’ says Hyung Jin, who specialises in machine learning, computer vision and human-robot interaction. ‘I’m mostly interested in human attention – trying to apply human attention mechanism to enhance computer vision tasks. That involves learning about the surroundings of the human.
‘Humans recognise what is seen as it is, but in many cases they also attend, identify and learn what is behind what is seen – in the environment or in the essentials of the object. Attending and understanding the motion characteristics and structure of articulated objects – from a complex object to a human body – through visual observation is one of the higher abilities human beings can perform, and this is one of the most difficult tasks that robots must ultimately achieve.
At Imperial College London, where he spent nearly five years as a post-doc before coming to Birmingham in January 2018, Hyung Jin’s main interest was articulated structure. ‘My main goal of my work is to enable the robot to live in its surroundings, just as humans do. I want to make them more human-like.'
Kinematic structures are crucial to humanoid robot development because they contain skeleton information, and also provide motion-related information between body parts. In his paper, Hyung Jin presents a novel framework for unsupervised kinematic structure-learning of complex articulated objects from a single-view 2D image sequence.
It addresses issues such as how to make a robot able to estimate the articulated object’s pose and how to enable it to find correspondence between two kinematic structures, such as those between a human hand and a robot hand. A typical scenario could be learning how to manipulate an articulated object or tool from a human’s demonstration – a task that requires the intelligence to understand human body movement, estimate kinematic structure of the object and learn characteristics of each movement joint and movement consequences.
‘This paper is about visually estimating the core of the kinematic structure – making the plausible and reasonable kinematic structure of something the robot knows nothing about by the iterative process of merging skeletal topology and motion information,’ says Hyung Jin. ‘Once you have achieved this visually-learning, latent kinematic structure of arbitrary objects – including the human body and the robot body itself – and corresponding kinematic structures between different objects, then someday the robot might be able to learn daily skills simply by observing us.’