Emerging trends in automotive sensing involve the development of fully autonomous vehicles to enhance environment perception, helping drivers and road users navigate safely. This will be done by integrating sensors with vehicle control systems, road infrastructure, through connectivity.
Radar is a prime sensor to deliver vehicle situational awareness in harsh environmental and lighting conditions, complementing optical sensors (video, LIDAR) which would not produce usable imagery the rain, spray, snow or darkness.
Just imagine you are driving on a motorway with 70m/h in the rain, and there is surface water ahead, on which you could slip and end up in the ditch. Will your eyes or video sensor see it through the rain and road spray, likely not, while radar can do, but this capability was never a mainstream of automotive research. Our goal is to develop new kind of cognitive imaging radar able to produce dense image of the whole road, and not only moving actors around, and we use:
- AI-based approaches (image segmentation and classification with features tracking).
- Sub-THz frequencies, able to deliver very fine range resolution and contrasting returns from all areas of different texture and roughness, so we can distinguish between water, grass and asphalt.
- Synthetic aperture techniques able to improve cross range resolution of our imagery.
At MISL, we aim to develop sensors and signal-processing algorithms to meet the requirements of the next-generation automotive sensing. This combines our mm-wave and sub-THz sensing suites with reliable platform kinematics to provide vehicles with the ability to sense the surface around them, allowing car systems to navigate safely through any type of surface. These frequency bands (77 GHz, 150 GHz and 300 GHz) are able to penetrate through the complex scattering media and discriminate different road surfaces, providing pre-emptive automatic measures for potentially dangerous conditions of the driving surface.
We are also designing sensors which will allow drivers to “see” the depth and tilt angle of a vehicle in water and control its speed over ground. Optimum vehicle progress control offers environmental benefits by reducing energy wastage by inappropriate driving and reduces damage to the terrain.
Our novel holistic simulator is used to understand radar-environment interaction, developing pedestrian and collision avoidance systems, establishing the key operational parameters, investigating effects of rain and spray on the radar signatures of vehicles, pedestrians, animals and bicycles.
Automotive imagery: SAR and Doppler beam sharpening
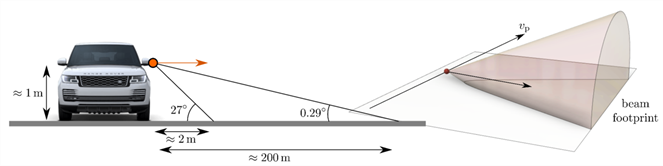
To improve cross-range resolution we developed SAR and Doppler beam sharpening (DBS), or unfocussed SAR, approaches to improve cross range resolution.
SAR, DBS and MIMO-SAR were the first obtained at MISL to demonstrate advantages of automotive imaging radar.
Multi-modal sensing with distributed radar network
One of the key requirements for autonomous driving and advanced driver assistance systems is 360-degree imagery of vehicle surroundings to ensure reliable scene mapping, situational awareness, path planning, and collision avoidance. To achieve this, we are developing a distributed radar sensor networks and developing reliable multi-modal imagery algorithms. MIMO radars are popular due to their ability to provide high resolution. Though their equivalent one-way beamwidth results in higher side lobe level, impeding dynamic range and ability to detect close targets, we have successfully combined MIMO with Doppler beam sharpening to lower side lobe level whilst improving resolution.
Image interpretation, segmentation and surface identification
We are conducting research on using the signal processing to extract the features of the road ahead, and from this, to interpret the road obstacle images in terms of size as well as positive or negative elevation. The developed techniques allow detecting and interpreting objects such as humps, kerbs, debris on the road tarmac, off road terrain profile and potholes on the road surface.
For safe navigation through any terrain we have developed a pixel-by-pixel description of the imaged scene (radar map) around the vehicle using image segmentation of the radar imagery.